Chat#
SquirroGPT is a Retrieval Augmented Generation (RAG) application powered by an underlying large language model (LLM). It is the enterprise-ready generative artificial intelligence (GenAI) application geared toward organizations searching for a natural language tool to conversationally interact with their data. Equipped with robust AI guardrails and privacy layers, the system meets the strict data security and privacy requirements of modern business standards.

In simpler terms, SquirroGPT is a digital assistant that uses cutting-edge artificial intelligence (AI) technology to allow you to securely and privately chat with your organizational data or with your website content through an embedded digital assistant. It provides sources for its answers, allowing you to quickly understand the context by navigating a wide range of document types directly within the application.

Also offered as an embedded digital assistant, SquirroGPT opens in your browser side panel, giving you constant access to all chat capabilities while navigating other websites.

Additionally, you can integrate the web app as an inline frame (iframe) inside other web pages.
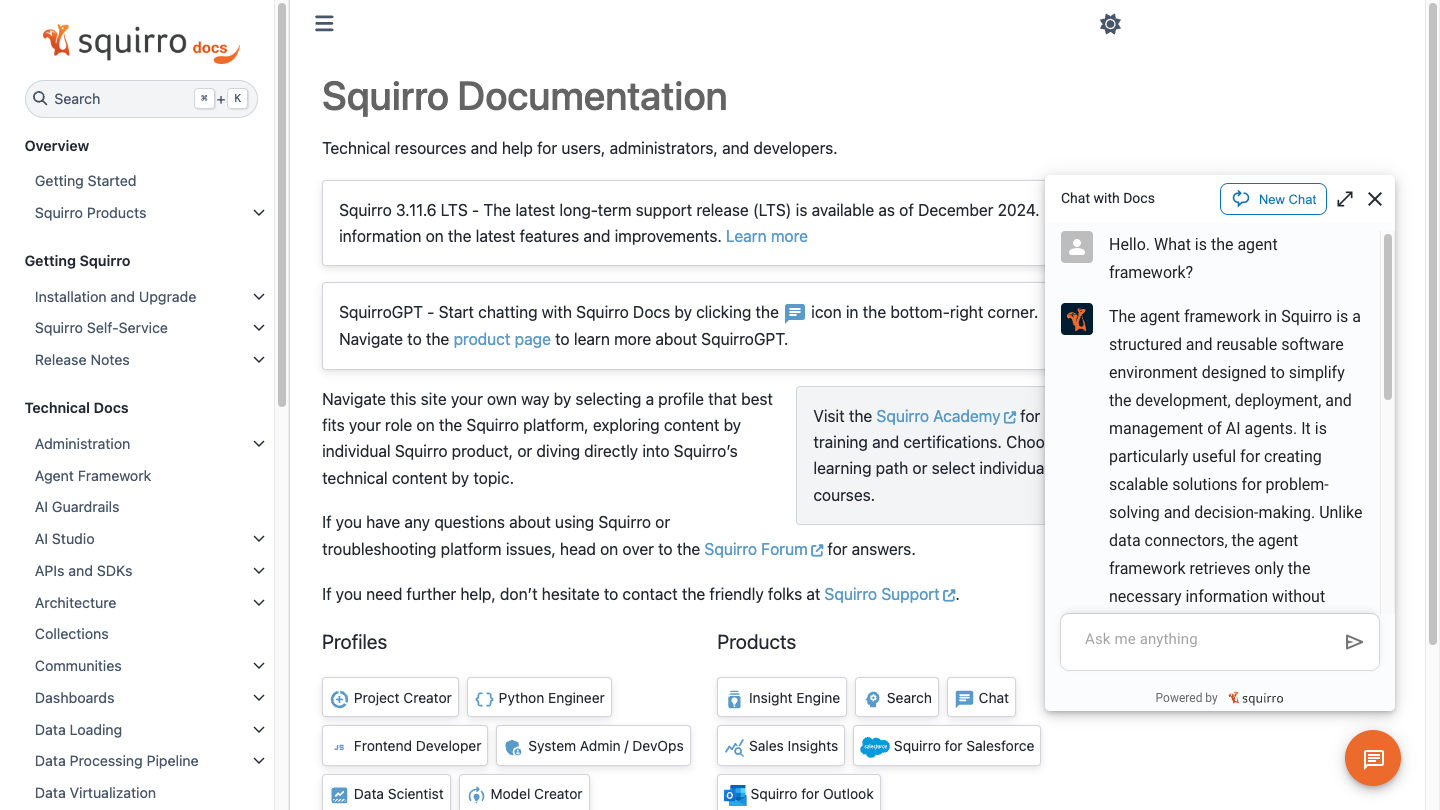
Personal Agents#
Using Personal Agents, you can engage in conversations with a large language model in various ways. The default mode takes all the project data into account, but to focus on specific areas, you have several options. You can chat with predefined selections generated from search results, which enables you to explore specific topics or questions. Alternatively, you can use collections of documents related to a particular subject to limit the scope of the discussion. Another approach is selecting favorite filters to narrow the conversation to a specific area of interest. Alternatively, for a more flexible and open-ended conversation, you can engage directly with the LLM to explore a wider range of topics, ask questions, generate text, or summarize content, using it as a versatile tool to support various tasks and objectives.

The Squirro administrator controls the availability of default agents, which come with every instance, and may choose to turn some of them off. Your organization selects a specific configuration, which determines the list of available agents. The Squirro administrator also designs and deploys custom agents tailored to meet your organization’s unique needs, providing a more personalized experience.
Configuration#
SquirroGPT is a powerful and user-friendly application designed to streamline your workflows with minimal setup required. It comes preconfigured with a carefully selected set of parameters, ensuring optimal performance right out of the box. To change these default settings, Squirro offers a web interface to control the configuration service, making it easy to adjust and fine-tune those parameters. The server-level settings ensure that all projects have the same parameters by default. The project-level settings, when configured, take precedence over the server-level ones.
Managing Agents#
Squirro administrators can create new agents and manage existing ones, allowing for a high degree of customization and control. When creating an agent, they assign a name for easy identification, can add a tooltip to help users understand the purpose and functionality, and have the option to add a visual element, such as an icon or picture, to enhance its presentation in the user interface. They also select the grounding, which determines whether it works with or without project data. Administrators can also reorder the list of agents as needed, to prioritize or organize them in a specific way.

Persona#
When managing an agent, the administrator can assign a specific persona, which enables the system to return information in a unique manner or follow particular instructions. The persona set at the agent level takes precedence over the one configured at the project level.

Query#
The query field functions similarly to any other search or query field, allowing you to refine and narrow down the scope of the discussion. By using this field, you can easily focus on, for example, a specific data source, company, product, or country.

Improving Conversations#
Every conversation with SquirroGPT starts with a prompt, a text input provided to the system that contains one or several questions, statements, or instructions. Here are some tips to help you get the most out of your SquirroGPT experience:
Wording#
The closer you word your prompts to how your connected documents are worded, the better your results will be.
If you know your documents talk about stock earnings in terms of “quarters”, use the term “earnings per share”, and generally use company stock symbols instead of names, here are a few recommended and not recommended prompts as examples:
What was Tesla’s stock earnings?
What was Tesla’s earnings in the last period of last year?
What was TSLA earnings per share in the fourth quarter of 2022?
Specificity#
The best prompts are detailed and specific to content that can be found (or suspected to be found) within the project’s connected documents.
Being detailed and specific also increases the likelihood of SquirroGPT returning quality sources.
If you are wondering if Squirro has any professional certifications, for example, it is better to be specific in your wording, as the following chat demonstrates:
Persona#
In the field of natural language processing (NLP) and artificial intelligence, prompt engineering refers to the design and optimization of prompts to help effectively interact with language models. Changing the persona setting helps you create an initial system prompt composed of characteristics and guidelines for the role SquirroGPT must adopt during a conversation.
You can change the default project-level persona using the project configuration wizard accessible from the chat widget. Click on the action menu in the bottom left corner and click Project Configuration.

Navigate to the Persona tab and add your persona instructions. Once saved, SquirroGPT automatically takes into account the new persona for the entire project, creating a flow of relevant, valuable, and consistent outputs for the target audience.
Example of the persona of a customer service representative:
You are a friendly and helpful customer service representative for an insurance company based in Switzerland. Your job is to answer customer inquiries truthfully. If you are unable to answer a query, advise the customer to contact the customer service department directly. Generally, when you find it challenging to provide an answer to a question, your first course of action should be to suggest a revised version of the question to the customer. If that is not feasible, then recommend that the customer provide additional context or ask a more specific question as a secondary option.
Project administrators can also change the persona using the Settings tab inside the Setup space.
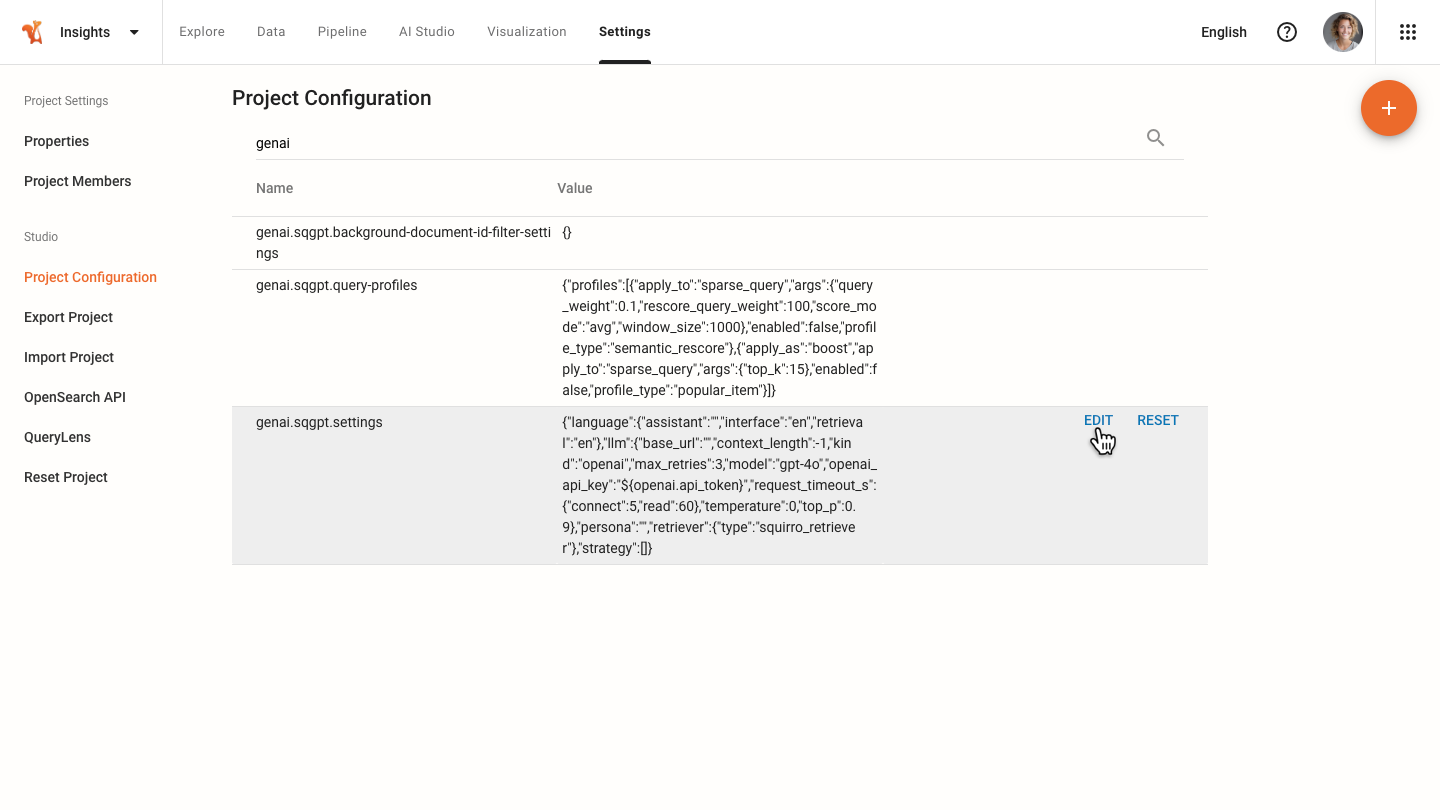
Note that the system combines the project-level persona with a system-level persona that comes preconfigured with every Squirro instance deployment. You can adjust that underlying persona to serve as the common foundation to support the various use cases of individual projects.
Suggestions#
The following is a list of prompt techniques that can be used to improve the quality of SquirroGPT responses.
You can ask SquirroGPT to explain answers in ways that appeal to different audiences and levels of precision.
This includes the following:
Explain in detail
Explain like I’m 5
Explain with examples
You can ask SquirroGPT to provide answers in a specific style. This is an alternative to the Explain technique.
Try the following:
Answer in a formal style
Answer in an informal style
Answer in descriptive style
You can request SquirroGPT to provide answers in a specific format, although not all formats supported by the underlying large language model are necessarily compatible with SquirroGPT.
Try the following:
Format the answer as a list
Format the output as HTML
Format the answer as a table
How It Works#
The following diagram illustrates how SquirroGPT processes user prompts using a RAG approach, where the system retrieves relevant source candidates and interacts with the underlying LLM to generate accurate answers.


Considerations#
SquirroGPT is a powerful tool, but like all generative technology powered by LLMs, it has its limitations, including the following:
Sources - Squirro instructs the LLM to identify and indicate the specific snippet of information provided used to generate an answer. However, the LLM may not always respond to this request, and when it does, the accuracy of the source attribution is not guaranteed. That limitation is inherent to LLM-powered applications and outside of Squirro’s control. There is no way to ensure that the LLM returns sources or that they are 100% accurate when it does.
Highlighting - For the same reason presented for sources, highlighting is not always 100% accurate. Additionally, a known issue with the PDF viewer prevents highlighting from being applied in multiple areas of documents.
Complex Charts and Visuals - SquirroGPT is not designed to accurately extract information from complex charts, infographics, or other visual elements.
Lengthy user prompts - If there is information SquirroGPT needs to query, that text should be uploaded as a document, not pasted into the chat.
Code snippets - Code snippets are not always produced accurately. The more bespoke the code, the larger the probability of issues.
Although some of the following features may sometimes work, they are not officially supported.
Transformative outputs - While SquirroGPT can provide basic formatting when it answers, it is not designed to transform documents into other formats or for other use cases, such as “Convert this document into a set of RFI responses”.
Multi-language and translations - While SquirroGPT may provide good translations or accurate answers in non-English languages, English is the only officially supported language.
SquirroGPT aims to provide accurate answers, but like other language models, it can occasionally generate responses not grounded in the provided sources, answers known as hallucinations. To ensure accuracy, Squirro recommends verifying SquirroGPT’s answers against the original sources. If you encounter an error, please use the feedback mechanism to report it to your Squirro administrators. Your input will help refine SquirroGPT and improve its response accuracy over time.
Getting Started#
Quickly explore Squirro chat capabilities with the Test Drive feature. Upload your data or crawl your website securely to see SquirroGPT in action. Learn more